1. 文献信息:题目——发表期刊/会议名称——发表年份;
Automated facial video-based recognition of depression and anxiety symptom severity: cross-corpus validation--Machine Vision and Applications --2019
2. 摘要内容是什么?(要理解人家如何写摘要的)
There is a growing interest in computational approaches permitting accurate detection of nonverbal signs of depression and related symptoms (i.e., anxiety and distress) that may serve as minimally intrusive means of monitoring illness progression. The aim of the present work was to develop a methodology for detecting such signs and to evaluate its generalizability and clinical specificity for detecting signs of depression and anxiety. Our approach focused on dynamic descriptors of facial expressions, employing motion history image, combined with appearance-based feature extraction algorithms (local binary patterns,histogram of oriented gradients), and visual geometry group features derived using deep learning networks through transfer learning. The relative performance of various alternative feature description and extraction techniques was first evaluated on a novel dataset comprising patients with a clinical diagnosis of depression (n = 20) and healthy volunteers (n = 45). Among various schemes involving depression measures as outcomes, best performance was obtained for continuous assessment of depression severity (as opposed to binary classification of patients and healthy volunteers). Comparable performance was achieved on a benchmark dataset, the audio/visual emotion challenge (AVEC’14). Regarding clinical specificity, results indicated that the proposed methodology was more accurate in detecting visual signs associated with self-reported anxiety symptoms. Findings are discussed in relation to clinical and technical limitations and future improvements.
3. 拟解决的问题(Motivation)
Limited continuous monitoring of persons at risk (e.g., individuals with a history of mental illness or suffering from chronic, debilitating physical diseases) is one of the factors contributing to the high rate of underdiagnosed depressive episodes [5].
对高危人群(例如,有精神病史或患有慢性身体衰弱性疾病的人)进行有限的持续监测是导致诊断不足的高比率抑郁发作[5]的因素之一。
4. 解决方法(Method)
Our approach focused on dynamic descriptors of facial expressions,
employing motion history image, combined with appearance-based feature extraction algorithms (local binary patterns, histogram of oriented gradients), and visual geometry group features derived using deep learning networks through transfer learning. The relative performance of various alternative feature description and extraction techniques was first evaluated on a novel dataset comprising patients with a clinical diagnosis of depression (n = 20) and healthy volunteers (n = 45). Among various schemes involving depression measures as outcomes, best performance was obtained for continuous assessment of depression severity (as opposed to binary classification of patients and healthy volunteers). Comparable performance was
achieved on a benchmark dataset, the audio/visual emotion challenge (AVEC’14).
我们的方法集中于面部表情的动态描述符,利用运动历史图像,结合基于外观的特征提取算法(局部二值模式,方向梯度直方图),以及通过迁移学习的深度学习网络获得的视觉几何组特征。各种替代特征描述和提取技术的相对性能首先在一个新的数据集上进行评估,该数据集包括临床诊断为抑郁症的患者(n = 20)和健康志愿者(n = 45)。在各种涉及抑郁措施作为结果的方案中,持续评估抑郁严重程度获得了最佳表现(相对于患者和健康志愿者的二元分类)。在一个基准数据集,音频/视觉情感挑战(AVEC’14)上取得了可比性的表现。
5. 实验结果及分析 (Results)
什么数据集,实验参数如何设置的,实验结果怎样?截图过来即可。
实验参数:
The study included two groups of participants: healthy volunteers (n = 45) aged 20–65 years without history of mental or neurological disorder, and patients suffering from MDD as diagnosed by their treating psychiatrists at the Psychiatry Outpatient Clinic, University Hospital of Heraklion (n = 20).
这项研究包括两组参与者:年龄在20-65岁之间、没有精神或神经疾病病史的健康志愿者(n=45),以及在伊拉克利安大学医院精神科门诊接受治疗的精神病学家诊断为MDD的患者(n=20)。
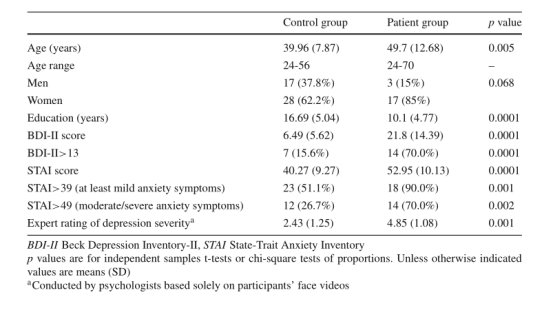
This took place prior to the description of positive experience/joy clip and, again, prior to the description of negative experience/sadness clip. Specifically, in the beginning of the protocol (c.f. Table 2 task #4) the participants were instructed by the research assistant on how to breathe in order to relax, while their heart rate and peripheral Blood V olume Pulse (BVP) were monitored through photoplethysmography using a NeXus-10 device (Mind Media, Netherlands). Participants were offered a second guided relaxation session immediately following the “Joy” video clip (Task #8 in Table2) to help them resume baseline levels of emotional and psychophysiological states (i.e., as recorded at the beginning of the experimental session). This was ensured by monitoring BVP on the Nexus-10 device during the breathing exercise. Facial video data used in the present study originated from steps 7–8 and 11–13 of the study protocol, shown in bold. The total duration of the experiment ranged from 60–90 min. A Point Grey Grasshopper®3 camera was employed to record high-resolution video at a high frame-rate. Camera settings were set to permit future assessment of the impact of recording quality on the algorithm efficiency. Benchmark tests showed that 80 frames per second (fps) and a resolution of 1920 × 1920 pixels was the maximum configuration that the available PC could support. Indirect lighting was applied totheparticipant’s facetoensureuniformfacialillumination and minimize shadows.
具体地说,在协议的开头(c.f.。表2任务#4)研究助理指导参与者如何呼吸以放松,同时使用Nexus-10设备(Mind Media,荷兰)通过光体积描记仪监测他们的心率和外周血液容积脉搏(BVP)。参与者在“快乐”视频剪辑(表2中的任务#8)之后立即接受第二次有指导的放松课程,以帮助他们恢复情绪和心理生理状态的基线水平(即,在实验课程开始时记录的)。这是通过在呼吸练习期间监测Nexus-10设备上的BVP来确保的。本研究中使用的面部视频数据来自研究方案的步骤7-8和11-13,以粗体显示。实验总时间为60~90min。使用Point Grey Grasshop®3摄像机以高帧率录制高分辨率视频。摄像机设置被设置为允许将来评估记录质量对算法效率的影响。基准测试显示,80帧/秒(Fps)和1920×1920像素的分辨率是可用的PC所能支持的最大配置。间接照明应用于参与者的面部,以确保面部照明均匀,并最大限度地减少阴影。
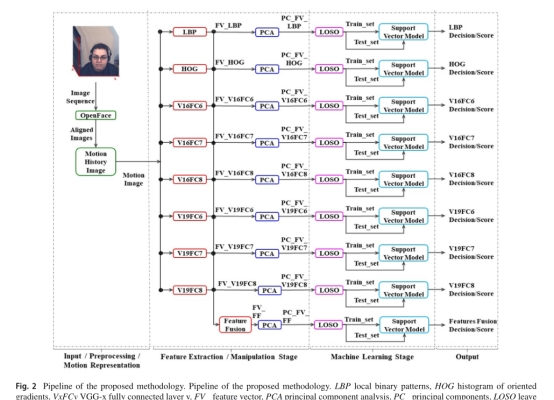
6. 总结/结论(Conclusions)
截图过来即可。
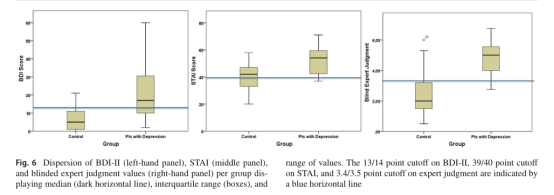
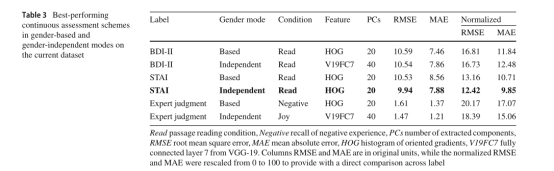
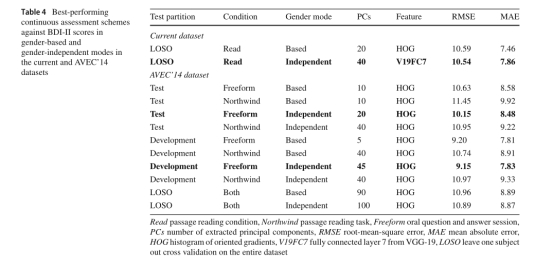
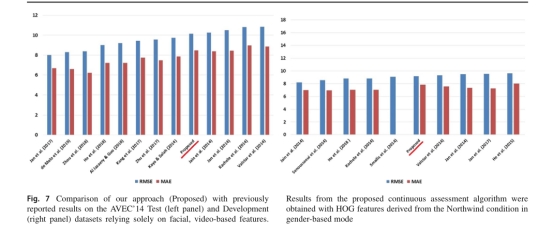
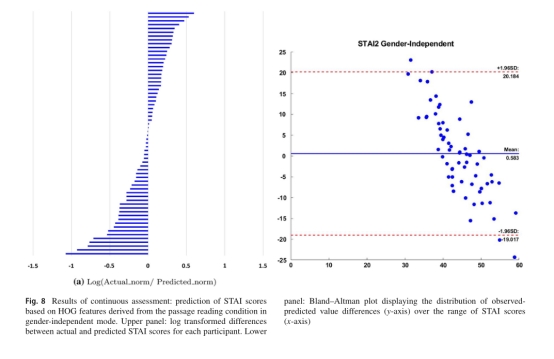
7. 还存在什么问题(Inspirations)
一般文章结尾处(conclusions and future work或discussion)可以找这个。
复制相关文字内容过来(不能截图)
For instance, a recent report of depression severity prediction using the Pittsburgh dataset relied on serial video recordings [56] whereas a single measurement was available in the current study. In other reports deep learning was employed for both algorithm training and tuning comparing [13,16] whereas in the current work this technique was used solely for feature extraction.
例如,最近一份使用匹兹堡数据集预测抑郁症严重程度的报告依赖于连续的视频记录[56],而在当前的研究中只有一种测量方法。在其他报告中,深度学习被用于算法训练和调优比较[13,16],而在当前的工作中,这种技术仅用于特征提取。
It should be noted, however, that best results (prediction of STAI or BDI-II scores) were obtained with video recordings fromtheneutral,passage-readingtaskingender-independent mode. As shown in the Bland-Altman plot of Fig. 8, t h e m o s t notable failure involved underestimation of STAI scores, given the higher associated clinical risk. Among the four patients in this category, two suffered from severe depression and were treated with high doses of anti-depressants, which may have affected the dynamics of facial expressions. Another patient spoke Greek as a second language and experienced some difficulty in reading the text, while the fourth patient also experienced some difficulty in reading due to reduced visual acuity. Clinicaldiagnosisofdepressiondidnotemergeasarobust out come variable in binary classification schemes .In part this findingmaybeattributedtotheconsiderableoverlapbetween the two study groups on self-reported depression symptomatology (BDI-II scores) and expert-rated facial signs of depression as shown in Fig. 6. The fact that the patients were
这种模式在实验条件下存在,因此可能反映了相对特征性的、动态的面部手势,尽管在情感和认知上没有挑战性的条件下(即阅读中性段落)获得的视频记录表现最好。然而,应该注意的是,最好的结果(STAI或BDI-II分数的预测)是通过中性的、阅读任务提供者无关的模式下的视频记录获得的。如图8的Bland-Altman图所示,考虑到相关的较高临床风险,显著的失败涉及对STAI评分的低估。在这类四名患者中,有两名患有严重抑郁症,并接受了大剂量的抗抑郁药物治疗,这可能影响了面部表情的动态。另一名患者将希腊语作为第二语言,在阅读课文时遇到了一些困难,而第四名患者也因为视力下降而在阅读方面遇到了一些困难。
8. 写作有什么特点:
8.1文章的整个结构包含那几个部分?
摘要,引言,数据收集,方法,结果,讨论
8.2引言(Introduction)撰写如何组织的?(找出“引言”中每一段话的中心句,或自己总结出中心句)
第1段:有中心句的话,截图过来即可。
这些工具既方便又经济,但有一定的缺点,因为它们没有考虑到个体特征、其他精神和医学并存(如焦虑症和症状)以及最近的主要应激源,而且容易受到有意或无意的报告偏见[4]的影响
第2段:有中心句的话,截图过来即可。
然而,考虑到目前最先进的技术水平,基于视频的抑郁症评估系统并不打算作为独立的工具,而主要是作为决策支持系统的一部分,帮助精神卫生专业人员对高危人群进行远程监控。考虑到大多数非语言的、面部的抑郁信号是动态的[4,7,8],几乎所有当前的方法都使用基于视频的特征,而不是基于帧(静态)的特征。
第3段:有中心句的话,截图过来即可。
几乎没有开放的数据集可用于支持新技术解决方案的开发,例如A VEC‘13-’141和DAIC-WOZ2数据集,它们是作为视听情绪挑战(A VEC)的抑郁识别子挑战(DSC)的一部分推出的。
8.3有什么语言表达优美的好句子可以摘录下来,供以后写作借鉴?例如,如何看“图”说话、看“表”说话、如何描述不同方法的性能高低和比较,如何表述因果关系。。。。等等。(摘录2-4个好句子)
好句子复制过来即可(不能截图)。
The A VEC dataset is suitable for assessing the generalizability of novel algorithms and analysis pipelines across cultures as it includes a range of video recording contexts and settings.
A VEC数据集适合于评估新算法和跨文化分析管道的通用性,因为它包括一系列视频录制上下文和设置。
The present study is addressing four specificaims.Firstly, to describe the development of a novel dataset comprising multimodal recordings from patients diagnosed with Major Depressive Disorder (MDD) and healthy volunteers. Data were obtained on a variety of experimental settings (including emotionally and cognitively neutral conditions and emotionally stimulating social and non-social contexts).
本研究致力于四个方面的研究:第一,描述一种新的数据集的开发,该数据集包括来自被诊断为严重抑郁障碍(MDD)的患者和健康志愿者的多模式记录。数据是在各种实验环境中获得的(包括情感和认知中立的条件以及情感刺激的社会和非社会环境)。
The generalizability of the developed method was tested on the A VEC’14 dataset, comprising 300 video recordings from 83 participants obtained in the context of two conditions which are very similar to tasks used in the present study, namely reading a neutral text passage and completing a question-and-answer session with the experimenter.
在A VEC‘14数据集上测试了该方法的概括性,该数据集包括83名参与者的300段视频记录,这些视频记录是在两个与本研究中使用的任务非常相似的条件下获得的,即阅读中性文本段落和完成与实验者的问答环节。