1. 文献信息:题目——发表期刊/会议名称——发表年份;
Visually Interpretable Representation Learning for Depression Recognition from Facial Images--TRANSACTIONS ON AFFECTIVE COMPUTING--2018
2. 摘要内容是什么?(要理解人家如何写摘要的)
Abstract—Recent evidence in mental health assessment have demonstrated that facial appearance could be highly indicative of depressive disorder. While previous methods based on the facial analysis promise to advance clinical diagnosis of depressive disorder in a more efficient and objective manner, challenges in visual representation of complex depression pattern prevent widespread practice of automated depression diagnosis. In this paper, we present a deep regression network termed DepressNet to learn a depression representation with visual explanation. Specifically, a deep convolutional neural network equipped with a global average pooling layer is first trained with facial depression data, which allows for identifying salient regions of input image in terms of its severity score based on the generated depression activation map (DAM). We then propose a multi-region DepressNet, with which multiple local deep regression models for different face regions are jointly leaned and their responses are fused to improve the overall recognition performance. We evaluate our method on two benchmark datasets, and the results show that our method significantly boosts state-of-the-art performance of the visual-based depression recognition. Most importantly, the DAM induced by our learned deep model may help reveal the visual depression pattern on faces and understand the insights of automated depression diagnosis.
Index Terms—Depression recognition, face recognition, deep
convolutional neural network, depression activation map.
3. 拟解决的问题(Motivation)
For the hand-crafted feature descriptors [22], [23], [24], [25], the extraction of effective depression features often involves a set of complicated image processing steps, and thus it depends heavily on the expertise knowledge. Most importantly, these low-level feature descriptors are not discriminative enough and hard to capture the high-level semantic structures encoded in depression faces.
The depression recognition methods discussed above are typically based on the hand-crafted image descriptors, which rely heavily on expert knowledge and are difficult to capture high-level semantic structures.
Nevertheless, these CNN-based depression recognition approaches are generally hard for clinical practitioners to understand the insight of depression feature learning and, most importantly, they cannot explain which region of the facial image is salient and discriminative for prediction of its severity level.
简述:使用传统的手工标签的方法需要经历复杂的步骤,这严重依赖于专家的经验,最重要的是这些低维度的信息非常难以区别,并且无法捕捉到藏在面部中的高维度的信息。
传统的CNN抑郁识别方法很难让医生专家知道抑郁特征,最重要的是,它不能解释哪一块面部图像的区域可以有效的预测抑郁水平。
4. 解决方法(Method)
In this paper, we propose a deep CNN architecture termed DepressNet, and derive a deep regression learning algorithm for visual depression feature learning and prediction of the depression severity level given a set of face images from a single subject in video. Figure 2 illustrates the pipeline of our DepressNet-based ADD scheme. For a video input to the DepressNet, face detection and cropping are first performed to obtained the three-dimensional face subvolumes. Then, these facial images are fed into the DepressNet for deep depression feature learning. Finally, the DepressNet outputs the depression score, as well as the salient regions in terms of its severity score.
使用了一种名为抑郁网络的深度CNN架构,推导出了一种深度回归学习算法用于学习视觉抑郁特征,并预测抑郁严重程度。图fig2演示了基于depression的ADD(automatic depression detection自动抑郁检测)方案管道。对于一个输入到抑郁网络的视频,首先对它进行人脸检测和剪裁,得到三维人脸ssubvolumes。然后这些面部图像被输入到抑郁网络中进行深度抑郁特征学习。最后,抑郁网络输出抑郁得分,以及严重程度得分的显著区域(也就是在面部图像中显示出特征部位的颜色)。
其他要点:
由于缺乏带标签的数据,CNN容易过拟合,所以用深度残差网络(deep residual network)ResNet50进行预训练
用GAP layer(不是FC层),与回归输出进行线性连接
如何找出显著区域:在我们的抑郁网中,最后一个卷积层的每个神经元都被其接受区特定的视觉抑制模式激活,而Ik特征图反映了这种视觉抑制模式的存在。DAM(depression activation map抑郁激活图)被认为是这些凹陷在不同图像协调下的加权总和。最后,通过将抑郁激活映射调整到输入面部图像的大小,我们可以找到显著的图像区域,这些区域根据抑郁严重程度的输出分数有区别。
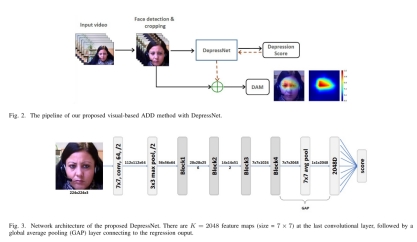
5. 实验结果及分析 (Results)
什么数据集,实验参数如何设置的,实验结果怎样?截图过来即可。
实验设置:
是欧阳Dlib包对每一帧进行人脸检测,调整大小为224x224,对AVEC2013(数据集)和AVEC2014分别从每100帧中抽取1帧和10帧进行实证提取。
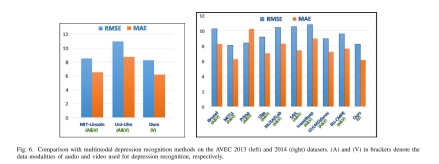
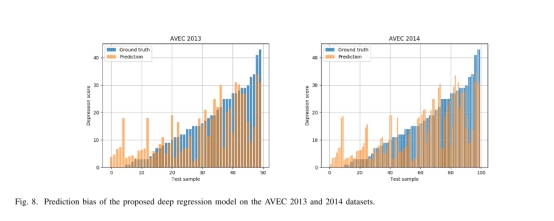
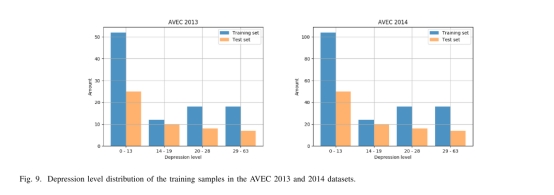
6. 总结/结论(Conclusions)
In this paper, we have presented a deep regression network called DepressNet to learn a depression representation with visual explanation that facilitates clinical prediction of depression severity from facial images. Specifically, a deep residual network equipped with a GAP layer was introduced to learn a deep regression model on the visual depression data, which not only facilitates accurate prediction of the depression severity, but also allows for identifying salient regions of the input facial image in terms of its severity level by generating the depression activation map (DAM). We also proposed a multi-region DepressNet, by which multiple local deep regression models for different face regions are jointly leaned and their responses are fused to improve the overall recognition performance. We evaluated our proposed method on two benchmark depression datasets, and the results showed that our method significantly boosts the state-of-theart performance of visual-based depression recognition. We believe the DAM induced by our deep regression model may help discover visual depression pattern on human faces and understand why the learned deep model works. We also observed in the experiments that the proposed deep
regression model may perform the conservative prediction in ADD. It means that the predicted depression score is often lower than the ground truth, especially for the cases that the patients are with minimal, moderate or server depression levels. The prediction bias can be intuitively observed from the Figure 8. One reasonable interpretation is that the training dataset has considerably larger number of normal facial images (with minimal depression level) than that of depressive facial images (with mild, moderate or server depression level). This imbalanced distribution of training samples in the two depression datasets is illustrated in Figure 9. In future work we are interested in investigating the depression recognition with multimodal depression data (e.g., audio, video, and facial dynamics) to further improve the overall prediction performance, although our proposed visualbased solution has achieved better performance than most existing multimodal solutions. Moreover, we plan to evaluate our method on the databases of other mental health institutions to consolidate the results as well as to enhance the learned deep depression representation that would benefit from being trained with a larger number of depression data.
结论简述了本实验的一些方法和方法的优缺点,本实验提出的抑郁网络可以带来一种视觉解释,有助于从面部图像预测抑郁症的严重程度。还提出了一种多区域抑郁网络,通过联合不同的面部区域并融合它们来提高识别性能。
后面还讲述了本实验的一些不足和未来的展望,比如研究多模态抑郁症数据(包含音频,视频和面部动态),后面还希望评估不同心理健康中心的数据来提高模型的性能。
7. 还存在什么问题(Inspirations)
一般文章结尾处(conclusions and future work或discussion)可以找这个。
复制相关文字内容过来(不能截图)
In future work we are interested in investigating the depression recognition with multimodal depression data (e.g., audio, video, and facial dynamics) to further improve the overall prediction performance, although our proposed visualbased solution has achieved better performance than most existing multimodal solutions. Moreover, we plan to evaluate our method on the databases of other mental health institutions to consolidate the results as well as to enhance the learned deep depression representation that would benefit from being trained with a larger number of depression data.
将来要研究多模态抑郁症数据(音频、视频和面部动态)的抑郁症识别,以进一步提高预测性能。计划在其他心理健康机构的数据库上评估本方法,以增强学习深度抑郁症表征。
8. 写作有什么特点:
8.1文章的整个结构包含那几个部分?
1. 题目
2. 摘要
3. 引言
4. 相关工作
5. 方法
6. 实验
7. 讨论和总结
8. 致谢
9. 参考文献
8.2引言(Introduction)撰写如何组织的?(找出“引言”中每一段话的中心句,或自己总结出中心句)
第1段:While proper antidepressant medication and psychological counseling can be helpful for treatment of MDD, diagnosis of MDD may be delayed or missed when the signs and symptoms are interpreted as normal moodiness. This issue is even more marked in developing countries.
第2段:With the increasing amount of people suffering from depression around the world, approaches to automated depression diagnosis (ADD) are highly desired to facilitate its objective assessment and efficient diagnosis
第3段:For the hand-crafted feature descriptors [22], [23], [24], [25], the extraction of effective depression features often involves a set of complicated image processing steps, and thus it depends heavily on the expertise knowledge. Most importantly, these low-level feature descriptors are not discriminative enough and hard to capture the high-level semantic structures encoded in depression faces. In contrast to hand-crafted descriptors, learning-based methods exploit some prior knowledge such as the sparsity [26], [27], compactness [28] and nonlinear hierarchy [29] for data-driven representation learning, leading to more accurate and robust recognition performance of videobased ADD [12], [14].
第4段:While very few attempts were made so far to develop deep
learning techniques for ADD, deep convolutional neural network (DCNN) based deep learning methods could potentially change the computing paradigm of the conventional visualbased ADD framework in two aspects.
第5段:Most importantly, the DAM induced by our learned deep model may help reveal the visual depression pattern on human faces, and understand the insights of visualbased depression recognition (i.e., why the learned deep model works), which has not yet been addressed in previous visualbased depression recognition.
8.3有什么语言表达优美的好句子可以摘录下来,供以后写作借鉴?例如,如何看“图”说话、看“表”说话、如何描述不同方法的性能高低和比较,如何表述因果关系。。。。等等。(摘录2-4个好句子)
1.With the increasing amount of people suffering from depression around the world, approaches to automated depression diagnosis (ADD) are highly desired to facilitate its objective assessment and efficient diagnosis
2.Most importantly, these low-level feature descriptors are not discriminative enough and hard to capture the high-level semantic structures encoded in depression faces.